Ministry of Science & Technology
Machine learning helps predict crustal movements in Tibetan Plateau
Posted On:
27 AUG 2024 2:46PM by PIB Delhi
Machine learning techniques used by scientists for modeling crustal deformations over the Tibetan Plateau have helped forecasting velocity vectors of such movements and enhancing the characterization of plate movements.
Typically, a dense network of Continuously Operating Reference Stations (CORS) is employed to continuously monitor crustal deformation. Campaign-mode GPS surveys are often used to densify the existing CORS network. Establishing a station in the desired location can be very challenging due to logistical problems and regional geographical considerations. Moreover, this process is expensive, and studies on crustal movement are often hampered by data gaps caused by logistical restrictions. Machine learning technique can come as succour in such situations to predict GPS site velocity for crustal deformation research.
To obtain velocity vectors at desired locations, scientist from Wadia Institute of Himalayan Geology, an autonomous Institute under the Department of Science and Technology (DST), Govt. of India, implemented machine learning techniques such as support vector machines, decision trees, and Gaussian process regression to accurately model crustal movement.
The scientists analysed data from 1,271 permanent continuous and campaign-mode GPS stations located on the Tibetan plateau and its surrounding areas. They used data from 892 stations for model training and data from 379 stations for testing.
The study published in Journal of Asian Earth Sciences demonstrates the effectiveness of these ML techniques in forecasting velocity vectors—easting velocity (VE) and northing velocity (VN)—and enhancing the characterization of plate movements. The correlation between the predicted and actual velocity vectors was found to be highly satisfactory making these ML predictive models considerably reliable for estimating geodetic velocity vectors.
Based on the data-driven trends from the existing trained models, the scientists fed the locations of arbitrary GPS sites and predicted the VE and VN at those locations.
The predicted velocities showed similar patterns to those obtained from neighbouring GPS stations. The ML algorithm demonstrates a remarkable achievement in the field of geodetic studies in a cost-effective manner.
Publication Link: https://doi.org/10.1016/j.jseaes.2023.106004
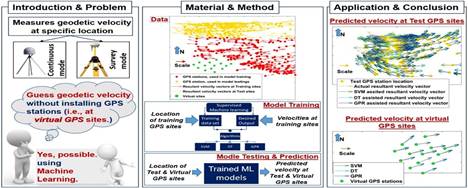
Fig. 1. The graphical abstract illustrates the problem statement, methods used, and their utilization, along with the practical implementation.
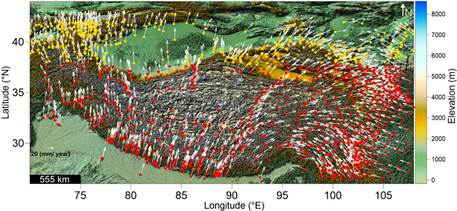
Fig. 2. Coordinates of GPS stations in the Tibetan Plateau and surrounding area. The length of the arrow denotes the magnitude of the resultant velocity vector, and the direction of the velocity vectors is measured by its angle from north. The position of the stations marked with red color are used for training, whereas the position of the stations marked with yellow color are used for testing.
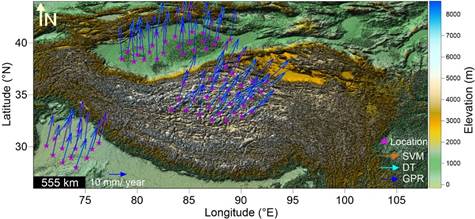
Fig. 3. Predicted velocity vectors at desired locations obtained from the SVM, DT and GPR predictive models.
***
KSY/PSM
(Release ID: 2049046)
Visitor Counter : 532