Ministry of Science & Technology
Amalgamated hybrid materials enable brain-mimicking artificial synapses for computing breakthroughs
Posted On:
06 FEB 2025 5:05PM by PIB Delhi
Taking inspiration from nature, a group of scientists have amalgamated hybrid materials to form a robust biomimetic system that closely mimics the behavior of biological synapses. This can pave the path towards a new wave of innovation in computing with breakthroughs in robotics, machine learning, and real-time data processing.
The human brain, known for its remarkable energy efficiency, is serving as a model for developing advanced technologies. At the forefront of these efforts are solution-processed memristor (a non-volatile electrical component that regulates the flow of current in a circuit) devices, which are designed to replicate the brain's synaptic functions. These devices are not only efficient but also scalable and cost-effective, making them ideal for creating neuromorphic systems-computers that function like the human brain. By mimicking how neurons communicate and process information, memristors have immense potential to revolutionize artificial intelligence, enabling smarter, faster, and more energy-efficient AI systems.
In a recent finding, a group of scientists from S. N. Bose National Centre for Basic Sciences (SNBNCBS), in collaboration with the National Institute of Technical Teachers' Training and Research (NITTTR), developed a hybrid material incorporating nanoscale conductive clusters to facilitate the formation of metallic pathways within a memristive layer. The foundation of the technology is the development of mesoporous graphitic carbon nitride (g-C3N4, abbreviated as CN) nanosheets embedded with silver nanoparticles (Ag NPs) to enable incremental resistance modulation via electric field-induced electrochemical metallization. The amalgamation of these hybrid materials by SNBNCBS, an autonomous institute of Department of Science and Technology, forms a robust biomimetic system known as AgCN that closely mimics the behavior of biological synapses. The research was published in the journal Advanced Functional Materials,
The AgCN system exhibits gradual and continuous changes in resistance, a property that is critical for energy-efficient and adaptive computing systems. The utilization of biomimicry principles in neuromorphic computing devices has yielded unparalleled capabilities. Contrary to conventional computing systems that employ rigid algorithms, neuromorphic systems emulate the brain's capacity for learning and adaptation. AgCN-based memristors exhibit remarkable versatility and adaptability in this domain.
These devices effectively replicated Morse Code by modulating their current to produce precise dot-and-line signals, underscoring their potential for real-time code detection applications. The core innovation of this technology lies in the electric field-induced strengthening or weakening of metallic pathways through the conductive clusters, which play a crucial role in modulating synaptic plasticity.
The devices can learn, adapt, and detect patterns with remarkable accuracy by varying voltage pulse numbers, amplitudes, and widths. A particularly noteworthy demonstration involved the devices' capacity to emulate Pavlov's dog experiment, thereby highlighting their aptitude for associative learning, a process analogous to biological learning. The impact of these devices extends beyond mere imitation of synaptic behavior, as they empower machines to learn and adapt with greater efficiency by processing and transmitting information in a manner analogous to biological synapses.
This capacity is of paramount importance for next-generation AI systems, which necessitate high-speed, low-power solutions for image recognition and real-time decision-making tasks. The development of conductive-island-assisted synaptic devices represents a significant leap forward in the field of artificial intelligence, as biomimicry drives innovation.
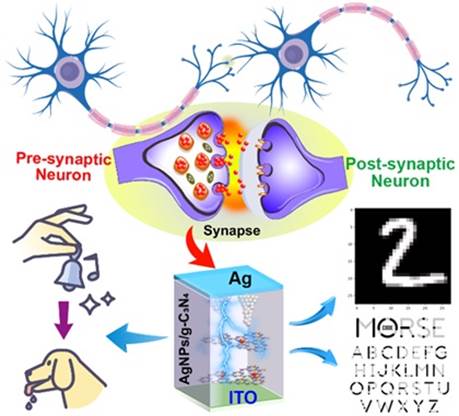
***
NKR/PSM
(Release ID: 2100327)
Visitor Counter : 419